Building Scalable and Efficient Software Architectures
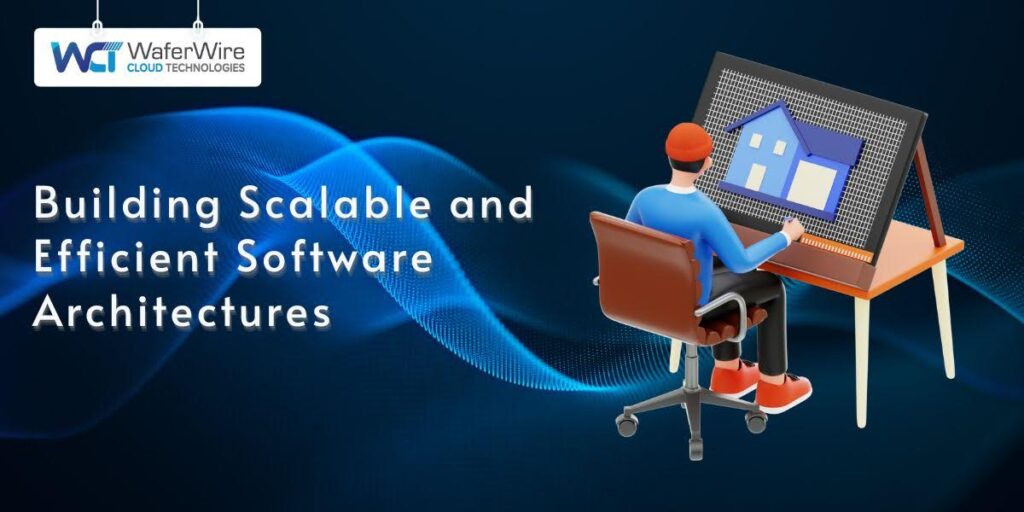
The need for scalable systems has become a fundamental requirement for businesses to stay competitive and agile in the contemporary digital world. For organizations looking to optimize their operations, scalability allows them to adapt to changing needs, efficiently manage resources, and ensure smooth performance during fluctuations in user activity. In this article, we will explore the key principles behind scalable architecture, practical strategies to avoid bottlenecks, and how you can build systems that grow with your business. Whether you’re aiming to streamline your infrastructure or enhance customer experiences, understanding scalability is crucial for future-proofing your operations. Through this blog, you’ll gain insights into building systems that can scale effortlessly, ensuring long-term business growth and operational success. Understanding Scalability Scalability refers to the ability of a system, network, or process to handle increasing workloads by adding resources in a way that maintains performance and efficiency. It ensures that businesses can respond effectively to growth, whether in user base, transactions, or data processing demands. For example, during a holiday sale, an e-commerce platform with scalable architecture can accommodate traffic spikes without downtime or slow performance. This adaptability not only enhances user experience but also safeguards revenue and reputation. With this understanding, let’s dive into the principles that form the foundation of scalable systems. Principles of Scalable Architecture Designing a scalable architecture requires adhering to foundational principles that ensure flexibility, performance, and resilience. These principles guide the creation of systems that can adapt to changing needs without compromising efficiency. By adhering to these principles, organizations can build systems that are both robust and adaptable. Let’s now explore the patterns commonly employed in scalable architectures. Common Patterns in Scalable Architecture To achieve scalability, businesses adopt architectural patterns that promote efficiency, flexibility, and performance under varying loads. Up next, we’ll compare the two primary scaling methods: vertical and horizontal scalability. Vertical vs. Horizontal Scalability Scalability strategies are broadly categorized into vertical and horizontal methods, each offering distinct advantages and challenges. Aspect Vertical Scaling Horizontal Scaling Definition -Adding more resources (e.g., memory, CPU, storage) to a single server to enhance capacity. -Adding more servers or machines to distribute the workload. Advantages – Simpler implementation and management.- Ideal for applications with limited scaling requirements. – Offers better fault tolerance and flexibility.- Suitable for high scalability demands. Limitations – Physical and cost constraints due to hardware upgrades. – Requires sophisticated system management and load balancing. Best Use Case – Useful for small-scale systems with predictable workloads. – Optimal for large-scale systems or environments with fluctuating demand. Scalability Cap – Limited by hardware constraints (finite scaling). – Virtually limitless with the ability to add more servers. WaferWire has helped numerous organizations transition from vertical to horizontal scalability, leveraging cloud technologies to ensure cost-effective and future-ready solutions. With a proven track record, WaferWire can guide your journey to a more scalable and efficient data infrastructure. Now, let’s explore how cloud computing takes scalability to the next level. Leveraging Cloud Computing for Scalability Cloud computing has revolutionized scalability, offering on-demand resources and pay-as-you-go models that cater to businesses of all sizes. From cloud benefits, we now move to design best practices that further enhance scalability. Best Practices for Designing Scalable Systems Designing scalable systems involves adopting best practices that ensure reliability, efficiency, and adaptability. To maintain scalability, it’s essential to proactively address potential bottlenecks. Avoiding Scalability Bottlenecks Scalability bottlenecks can hinder system performance and user experience. Proactive measures can prevent such issues. Now, let’s take a closer look at Azure Data Infrastructure and how it serves as a prime example of scalable architecture in action. Azure Data Infrastructure for Scalability and Resilience When designing a scalable architecture, leveraging a robust cloud-based data infrastructure is crucial. Azure Data Infrastructure serves as an industry-leading example, offering businesses the ability to store, process, and manage data efficiently while ensuring high availability and security. Here are some key benefits of Azure Data Infrastructure: By leveraging Azure’s scalable data infrastructure, businesses can build resilient, future-proof architectures that drive efficiency, agility, and innovation. Conclusion Scalable architecture is a cornerstone of modern business success, enabling organizations to adapt, grow, and thrive in competitive markets. By implementing core principles like loose coupling, leveraging cloud computing, and adopting best practices, businesses can create systems that are both flexible and robust. WaferWire’s expertise in designing and deploying scalable architectures ensures that businesses are equipped to handle growth without compromising performance. Ready to future-proof your operations? Contact WaferWire today to explore scalable solutions tailored to your needs!
Understanding the Importance of Modernizing Your Data Estate
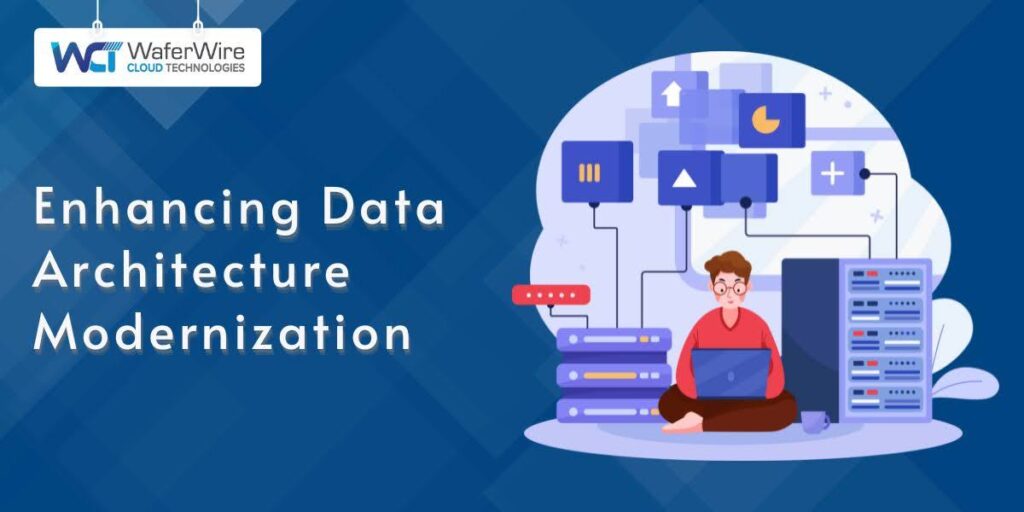
As industries become increasingly dependent on data, the importance of modernizing your data estate cannot be overstated. The ability to leverage data effectively offers a competitive advantage, enabling organizations to make informed decisions, and drive innovation. In this article, we will discuss the key reasons why data modernization is crucial, the benefits it brings, and how to implement a robust strategy for success, helping your organization stay competitive, improve decision-making, and unlock new growth opportunities. Recognizing the Critical Role of Data Modernization Modernizing your data estate involves a fundamental shift from legacy systems to more agile, scalable, and efficient data environments. This transformation is pivotal to unlocking the full potential of your data. Let’s explore why modernizing your data estate is crucial for staying competitive and adaptable in evolving business landscape. With a clearer understanding of why data modernization is crucial for your organization, let’s now explore the specific benefits that come with upgrading your data estate. Benefits of Modernizing Your Data Estate Data modernization brings a range of tangible benefits that positively impact your organization’s bottom line. Let’s take a closer look at the numerous benefits that come with this strategic move. These advantages can significantly enhance both your organization’s operations and its financial health. By modernizing your data estate, your organization not only improves performance and cost efficiency but also gains a future-ready foundation for AI-driven innovation. Next, let’s explore the key components that form the backbone of a modern data estate. Core Components of a Modern Data Estate The foundation of any modern data estate involves several critical components, each of which plays a crucial role in ensuring seamless data management and accessibility. To ensure your data is optimized for modern environments, it is important to understand the core components that make up a data estate. These components provide the necessary infrastructure to manage, analyze, and store your organization’s data effectively. With a clear understanding of the core components of a modern data estate, let’s now look into the data management practices that help ensure optimal operation and compliance. Modern Data Management Practices To stay ahead in the market, businesses must implement best practices in data management to protect their data assets and maximize their value. After understanding the core components of a modern data estate, it’s important to explore the management practices that enhance data security, governance, and compliance, ultimately supporting better decision-making and operational efficiency. As we have covered the best practices for managing modern data estates, let’s now discuss the steps required to effectively implement data modernization within your organization. Strategies for Implementing Data Modernization Successfully modernizing your data estate requires a strategic approach that aligns with your organization’s goals and resources. With the groundwork of benefits, core components, and best practices laid out, we can now focus on how to effectively implement a modernization strategy within your organization. This is where careful planning and leveraging the right tools will help ensure the success of your modernization efforts. To ensure effective data modernization, careful planning and execution are essential. Neglecting data estate modernization can lead to significant risks, which we’ll explore next. Risks of Neglecting Data Estate Modernization Failing to modernize your data estate can have serious consequences, from performance bottlenecks to increased costs. It is important to recognize these risks and take proactive steps to avoid them. Let’s explore the specific risks associated with neglecting this crucial process. Conclusion The modernization of your data estate is not just a technological upgrade—it’s a strategic move that positions your organization for long-term success in an increasingly data-driven world. By adopting modern tools, infrastructures, and best practices, you can enhance decision-making, and drive innovation. However, data modernization requires careful planning and execution to ensure your organization’s data is leveraged to its full potential. WaferWire is here to guide you through this journey, offering tailored solutions that support businesses in achieving a seamless and effective data modernization process.Don’t let outdated systems hold you back. Modernizing your data estate will give your organization the tools and flexibility it needs to stay competitive. If you’re ready to start your modernization journey or need expert advice, contact WaferWire today.
Designing a Modern Data Pipeline: From Scratch to Scalable Structure
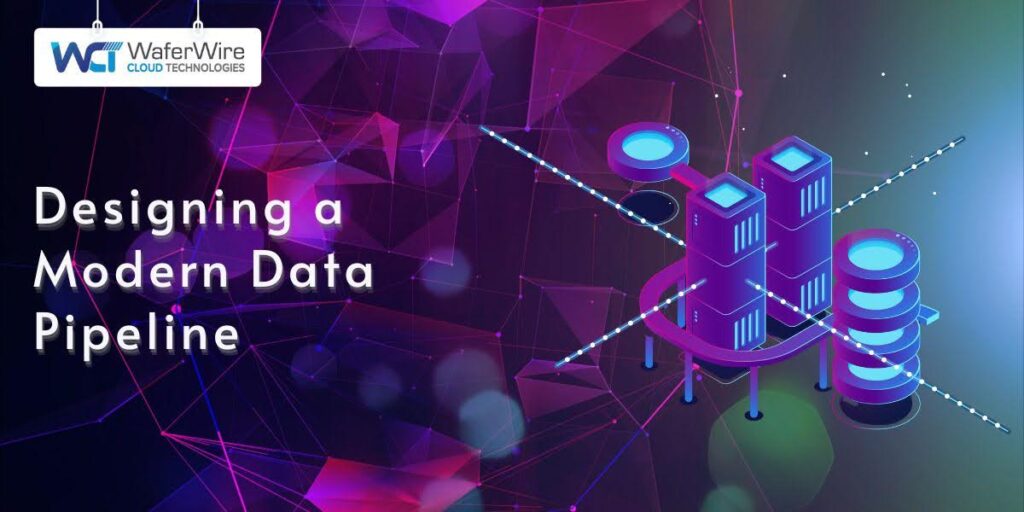
Organizations benefit significantly from building data pipelines that are efficient, scalable, and reliable, capable of managing the ever-growing influx of information. A modern data pipeline design is essential for unlocking the full potential of data, transforming raw information into valuable insights that drive informed decision-making and streamline business processes. By adopting robust, scalable pipelines, businesses can stay ahead of the competition and accelerate data-driven innovation. This blog will discuss the key principles and best practices for building a modern data pipeline that is not only efficient but also scalable to meet future demands. Understanding Modern Data Pipelines A modern data pipeline is a series of processes that automate the flow of data from one point to another, ensuring that data is collected, processed, stored, and consumed efficiently. The primary purpose of a data pipeline is to streamline data movement and transformation to enable timely, accurate decision-making. Modern data pipelines are designed to handle vast and diverse data sources, ensuring the smooth and consistent flow of information. They integrate various data systems, automating tasks like data extraction, cleaning, and transformation, while maintaining high levels of reliability and performance. Importance of Data Pipelines in Managing Data Flow Effective data pipelines ensure that data moves seamlessly from the source to the destination without interruptions. By managing the flow efficiently, businesses can ensure timely data processing, reducing delays in decision-making. Role of Data Pipelines in Data Transformation and Integration A modern data pipeline does more than just collect data; it processes and transforms raw information into meaningful insights. Data from various sources is integrated, cleaned, and enriched to be consumed by various stakeholders, whether for reporting, analytics, or machine learning purposes. With this understanding of what a modern data pipeline entails, we can now delve into the key components that make up an efficient and scalable pipeline. Key Components of a Modern Data Pipeline To build a reliable and scalable data pipeline, organizations must focus on the essential components that work in harmony. These components ensure data is properly captured, transformed, and made available for analysis, all while maintaining high levels of performance and compliance. Now that we’ve covered the key components, let’s discuss the building blocks required for making the data pipeline scalable to accommodate increasing data demands. Building Blocks for Scalability When designing a scalable data pipeline, it’s essential to break down its core elements to ensure future growth is well-supported. Let’s explore these building blocks one by one. Scalability in data pipelines is achieved by focusing on specific foundational elements that enable systems to handle growing volumes and complexities effectively. Below are the critical building blocks for ensuring a scalable data pipeline: 1. Architecture Design Choosing the right architecture tailored to your needs is foundational. A modular architecture, which allows individual components to scale independently, ensures adaptability and simplifies troubleshooting as your pipeline evolves. 2. Smart Data Management Effective data management involves robust data quality checks, validation, and cleaning mechanisms. These practices reduce inconsistencies and maintain data integrity, which is vital as data volumes grow. 3. Parallel Processing Utilizing parallel processing frameworks such as Apache Spark or Hadoop enables your pipeline to process large datasets efficiently. By dividing tasks across multiple processors, parallelism minimizes delays and optimizes resource utilization. 4. Optimized Data Processing Latency reduction is key to scalability. Optimizing data transformations and workflows helps ensure smooth processing as datasets grow in size and complexity. This includes leveraging distributed processing and caching strategies. 5. Real-Time Processing Modern businesses require real-time insights. A scalable pipeline prioritizes real-time data processing to handle live streams and enables quicker decision-making while adapting to changes instantly. 6. Fault Tolerance A fault-tolerant architecture ensures uninterrupted operation, even in the event of hardware or software failures. Techniques such as data replication, retry mechanisms, and automated failovers are essential to building resilience. 7. Monitoring and Alerting Comprehensive real-time monitoring and alerting systems are crucial for tracking pipeline health and performance. Tools like Prometheus or Grafana provide insights into potential bottlenecks and ensure proactive issue resolution. 8. Cost Optimization Scalability must also be cost-effective. Designing pipelines with efficient resource allocation, leveraging pay-as-you-go cloud services, and reducing redundancies help manage costs while supporting growth. 9. Horizontal Scaling Scaling horizontally by adding more nodes or servers allows your pipeline to handle increasing data loads without performance degradation. This design enables flexibility and ensures consistent throughput under high demand. These building blocks form the foundation for creating a data pipeline that can effectively scale with your organization’s growing data demands. Let’s now look at best practices for ensuring these elements work together seamlessly to deliver high performance. Best Practices for Designing Scalable Pipelines When designing a modern data pipeline, applying best practices can ensure that the system is not only scalable but also reliable and efficient. Following these practices helps avoid common pitfalls and guarantees that the pipeline can evolve with the business. Design for Fault Tolerance and Resilience Scalability isn’t just about handling more data; it’s about ensuring that the system remains operational even when issues arise. Incorporating redundancy and failover mechanisms ensures that data continues to flow smoothly, even if one part of the system experiences issues. Adopt a Modular Approach A modular architecture makes it easier to scale different components of the pipeline independently. By breaking the pipeline into smaller, self-contained modules, organizations can focus on scaling only the parts that need it without overhauling the entire system. Automation to Streamline Processes Automation is key to reducing manual intervention and streamlining processes. By automating data cleaning, transformation, and movement, businesses can ensure that their pipeline operates efficiently at scale. Leveraging Cloud for Dynamic Scalability Cloud platforms provide the flexibility to scale infrastructure dynamically. With cloud-based solutions, organizations can quickly allocate resources as needed without having to invest in physical hardware. Ensuring Monitoring and Observability Real-time monitoring and observability are essential for maintaining the health of a data pipeline. By continuously tracking key metrics and setting up alerts, businesses can quickly identify bottlenecks or failures and take corrective action. After
Starting with Data Monetization Strategies: A Simple Guide
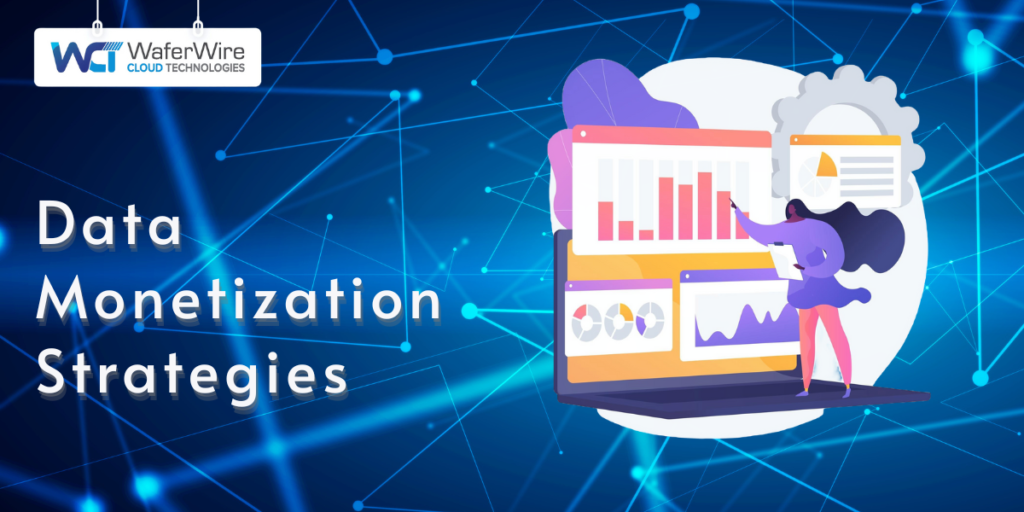
Businesses today are surrounded by data, customer preferences, sales trends, operational details—but most of it just sits there, unused. For decision-makers like CXOs and business leaders, the challenge is clear: how do we turn all this data into something valuable for our company? The truth is, figuring out how to use data effectively can be tricky. Your competitors are already using their data to improve products, save money, and grow faster, which means doing nothing isn’t an option. This guide is here to help you cut through the confusion. We’ll break down data monetization strategies into simple, practical steps. Whether in retail, manufacturing, financial services, or utilities, you’ll learn how to use your data to create measurable results. What is Data Monetization? Data monetization is the process of transforming a company’s data assets into direct or indirect economic value. It involves leveraging the data collected from various sources to create revenue-generating opportunities or operational efficiencies. Unlike general data utilization, data monetization focuses exclusively on converting data into measurable financial outcomes. At its core, data monetization is about recognizing the economic potential of raw data and converting it into value—whether through direct revenue, operational efficiencies, or strategic partnerships. Organizations use this process to unlock hidden opportunities, extend their market reach, and enhance their overall competitiveness. Key characteristics of data monetization include: Exploring the tangible benefits of data monetization reveals its importance for driving long-term business success. Benefits of Data Monetization Data monetization is more than adding to the bottom line; it’s transforming how businesses operate and grow. To fully capitalize on data’s potential, it’s essential to understand the different types of data monetization and how they work. Types of Data Monetization Monetizing data is a dynamic process with several pathways. Each strategy offers unique opportunities, from creating new revenue streams to enhancing business operations. The choice depends on how your data aligns with your business objectives. Below are some key types of data monetization. Data-as-a-Service (DaaS) This strategy involves providing raw, aggregated, or anonymized data to other businesses, typically in a direct business-to-business (B2B) model. DaaS requires minimal processing, as the data is often delivered as-is. Thus, the buyer is responsible for analyzing it using analytics or business intelligence (BI) tools. This method is ideal for businesses lacking internal analytics resources but with valuable datasets, such as customer trends or environmental data. While it’s straightforward to implement, its revenue potential is lower since raw data needs further processing to deliver value. DaaS is effective for organizations aiming to generate revenue while minimizing additional workloads. This method directly monetizes data and only needs basic data preparation. Insight-as-a-Service (IaaS) Unlike DaaS, Insight-as-a-Service offers processed and actionable insights, such as market trends or customer behavior patterns. These insights often result from analyzing internal and external datasets, making them more valuable than raw data. Businesses using this strategy typically provide reports, dashboards, or embedded analytics applications. The insights can be sold as one-time deliverables or subscriptions, creating recurring revenue opportunities. This approach demands a deeper understanding of the buyer’s needs and requires organizations to invest in data analysis tools and expertise. As a result, the pricing is higher than DaaS due to the added value of actionable recommendations. Analytics-as-a-Service (AaaS) AaaS provides buyers with access to analytics platforms and tools, enabling them to explore and analyze data independently. Unlike IaaS, AaaS offers real-time access to advanced analytics, BI visualization tools, and sometimes predictive models operated by the data provider. For instance, a market research company might allow buyers to interact with large-scale industry datasets through an intuitive analytics platform. This approach combines the benefits of providing both data and tools, offering a seamless user experience. AaaS is the most IT-intensive strategy and requires robust infrastructure to ensure data security and performance. While it carries a higher operational burden, it has significant revenue potential, particularly for businesses with large, dynamic datasets. Data-Driven Business Models This is an indirect monetization strategy that focuses on using data to improve internal processes, reduce costs, and enhance productivity. By leveraging operational and customer data, businesses can optimize decision-making across departments, such as sales, marketing, and supply chain management. For example, a retail business may use sales data to estimate demand and manage inventory levels, reducing overstock and improving cash flow. Similarly, IT departments can analyze server logs to discover reoccurring problems, lowering downtime and increasing service efficiency. This technique allows firms to generate value within rather than selling data externally, resulting in a sustainable paradigm for long-term growth. Each monetisation type serves different goals, from driving internal efficiency to creating external revenue opportunities. Next, we’ll examine how businesses can effectively implement these strategies. Approaches to Data Monetization Successful monetization relies on aligning data strategies with business objectives. The following approaches can help businesses turn data into measurable value: 1. Making Better Business Decisions Data allows companies to identify inefficiencies, uncover opportunities, and make informed decisions. By analyzing real-time data, logistics companies can optimize delivery routes, saving fuel costs while improving delivery times. Retailers use sales data to forecast demand and adjust inventory levels, reducing waste and increasing profitability. 2. Sharing Data with Partners Collaborating with vendors or third parties can create mutual benefits. Retail chains often share sales and inventory data with suppliers to streamline restocking processes. This partnership reduces stockouts, enhances supply chain efficiency, and ensures timely customer deliveries. 3. Selling Data as a Product or Service Organizations can package their data or insights into valuable products for external businesses. Telecommunications providers sell network usage trends to advertisers to refine targeting strategies. Financial institutions analyze spending habits to develop consumer behavior insights for corporate clients. Once you understand the approaches, the next step is building a solid foundation for data monetization. Key Steps to Start Data Monetization Turning data into a revenue stream starts with a solid plan. This section outlines a straightforward approach to help businesses launch their data monetization strategies effectively, ensuring measurable results and sustained growth. 1. Identify Potential Data Sources Your data estate is
Calculating ROI in Data Modernization Strategies
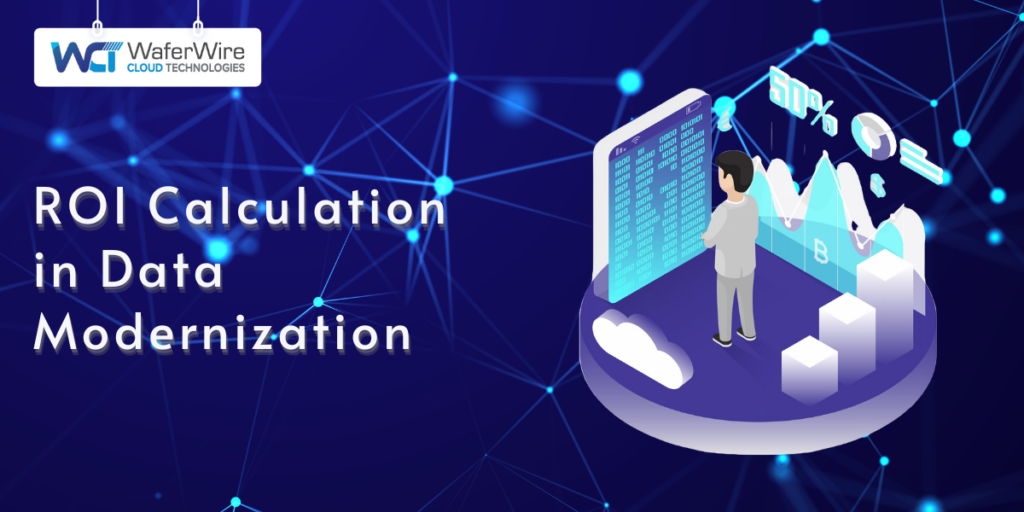
Measuring ROI in data modernization is essential for assessing the value gained from upgrading data infrastructure and processes. By focusing on measurable returns, businesses can align their modernization efforts with long-term strategic goals. In this blog, we will explore the importance of ROI in data modernization, key strategies to maximize it, and practical steps organizations can take to achieve sustainable success. The Importance of ROI in Data Modernization Understanding and prioritizing Return on Investment (ROI) is essential when planning data modernization initiatives. Modernization efforts that fail to yield clear and measurable results often lead to wasted resources and missed opportunities. ROI serves as a critical benchmark to evaluate whether the financial and operational improvements achieved through modernization justify the associated costs. It enables organizations to focus on the most impactful initiatives, ensuring resources are directed toward projects with the highest potential returns. By linking modernization goals to specific outcomes, businesses can prioritize areas where modernization creates the most value. Efforts like reducing data silos, automating workflows, and improving decision-making processes can significantly enhance ROI. Having established the importance of ROI, the next step involves understanding how to calculate it effectively to guide modernization efforts. Essential Steps to Calculating ROI Accurately calculating ROI requires a systematic and detailed assessment of current processes and their inefficiencies. This foundational step ensures that the benefits of modernization are measurable and directly tied to the organization’s strategic objectives. Strategies to Enhance Data Modernization ROI Achieving a strong ROI from data modernization requires adopting approaches that optimize both technology and processes. By leveraging modern tools and frameworks, businesses can unlock greater value from their data systems. Cost-Saving Practices in Data Modernization Cost optimization plays a central role in driving ROI, particularly for businesses seeking to modernize their data infrastructure without incurring excessive expenses. Streamlining data pipelines helps minimize resource consumption and improves process efficiency, ensuring that systems run smoothly without unnecessary expenditures. This optimization not only reduces costs but also enhances the scalability and flexibility of data systems. Beyond cost savings, optimizing the overall infrastructure is essential to achieve higher returns from modernization efforts. Optimizing Data Infrastructure for Higher ROI Modernizing the infrastructure that supports data operations is a crucial step toward ensuring sustained returns on investment. A well-optimized infrastructure enables organizations to scale operations effectively while maintaining high performance. Table: Optimizing Infrastructure Practices and ROI Impact Practice Benefit ROI Impact Integrated Data Management Streamlined operations and reduced delays Increased efficiency and faster ROI Metadata-Driven Insights Enhanced data reliability and accuracy Better decision-making and usability Automated Pipelines Reduced manual intervention and errors Lower operational costs Real-Time Analytics Faster access to actionable insights Quicker response to market demands AI-Driven Predictive Analytics Improved forecasting and proactive strategies Enhanced revenue growth opportunities AI-Driven Prescriptive Analytics Optimized decision-making with scenario evaluations Maximized resource utilization and ROI Scalable Cloud Infrastructure Seamless handling of increasing data volumes Cost savings and improved system agility Unified Data Governance Framework Compliance with regulations and reduced risk exposure Avoidance of penalties and improved trust To ensure continued success in modernization efforts, organizations must implement mechanisms to monitor and improve ROI consistently. Monitoring and Improving ROI Continuous monitoring and refinement of modernization strategies are essential to maintain and enhance ROI over time. This involves leveraging data-driven insights and predictive tools to adapt to evolving business needs. Training and Development Empowering teams to effectively use and maintain modernized systems is a critical component of achieving sustainable ROI. Investing in employee training ensures that technology is used to its full potential. Conclusion Aligning data modernization strategies with ROI goals is critical for achieving both immediate and long-term benefits. By focusing on measurable outcomes, businesses can justify investments, streamline operations, and position themselves for sustained growth. With tailored modernization solutions, WaferWire empowers organizations to confidently tackle the complexities of modernization while achieving maximum returns. As the data landscape continues to evolve, embracing strategic modernization approaches will be vital to sustaining business success. Ready to transform your business with expert guidance? Contact us today!
Enhancing Data Architecture Modernization for Business Success
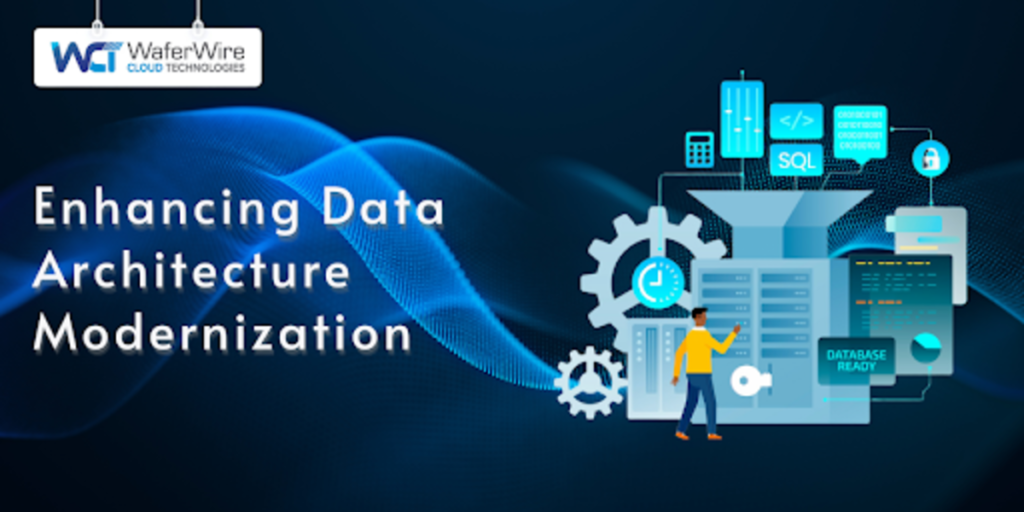
Data architecture modernization is a crucial step for businesses aiming to maximize the value of their data and future-proof their operations. As data volumes grow exponentially, outdated systems often limit an organization’s ability to manage and extract insights effectively. Transitioning to a modern, flexible, and scalable data architecture improves accessibility, streamlines workflows, and fosters innovation across teams. This transformation empowers businesses to make timely, well-informed decisions that drive strategic goals. In this guide, we delve into the importance of modernizing data architecture, explore its key components, and outline best practices for seamless implementation. Importance of Modernizing Data Architecture Modernizing data architecture is essential for organizations that want to optimize their data resources and keep pace with technological advancements. By updating your data systems, you lay the groundwork for efficient data management, better decision-making, and business growth. A modernized data architecture allows businesses to use data as a competitive advantage. It provides scalable, efficient systems that support faster decision-making and innovation. By leveraging advanced architectures, businesses can gain deeper insights, enhance customer experiences, and streamline operations, keeping them ahead of competitors. This approach helps organizations make the most of their data, driving growth and enabling them to adapt quickly to changing market demands. While modernizing data architecture brings numerous benefits, it often involves overcoming significant challenges. Companies must address legacy system integration, data silos, and ensuring data security. Despite these obstacles, modernizing data architecture creates a more agile, scalable system that meets future business needs. Modern architectures make data more accessible, organized, and actionable, enabling faster and more accurate decision-making. With high-quality data at hand, businesses can reduce risks and improve performance by relying on real-time insights. Now that we’ve established the importance of modernizing your data architecture, let’s dive into the key components that make up a modern data architecture. Key Components of Modern Data Architecture The key components of a modern data architecture are designed to work together to support scalable, flexible, and efficient data operations. 1. Data Lakehouse A data lakehouse combines the scalability of data lakes with the reliability of data warehouses. It allows organizations to store large volumes of raw, unstructured data while supporting advanced analytics and business intelligence. This hybrid model provides a single, unified platform for storing and analyzing both structured and unstructured data. 2. Data Fabric Data fabric connects disparate data sources, ensuring that data can be easily accessed across platforms. By integrating various data systems into a cohesive structure, data fabric breaks down silos, enabling organizations to make data more accessible to users across departments. 3. Self-Service Analytics Self-service analytics tools give business users the ability to explore data, create reports, and generate insights without needing deep technical expertise. This not only reduces dependency on IT teams but also fosters a culture of data-driven decision-making within the organization. With the key components outlined, let’s explore the core pillars that guide a modern data architecture’s effectiveness. Pillars of Modern Data Architecture Modern data architecture is built on several fundamental pillars that ensure its scalability, flexibility, and efficiency in handling complex data operations. 1. Interoperability Interoperability ensures that different data systems and platforms can work together seamlessly. By standardizing data integration practices, businesses can ensure that data flows smoothly across different environments, from on-premise systems to cloud-based platforms. 2. Decentralization Decentralization involves distributing data ownership across different business units, which is the core concept behind a data mesh. This approach allows each department to manage its data independently while ensuring that the data is available across the organization for seamless collaboration and insights. 3. Automation Automation is key to modern data architectures, as it ensures that repetitive data processes are handled efficiently. Automated workflows can help reduce human error, increase speed, and enhance the overall reliability of data systems, making them more adaptable and scalable. Now that we’ve covered the pillars of modern data architecture, let’s take a look at the challenges organizations face when trying to modernize their data infrastructure. Challenges in Modernizing Data Architecture Modernizing data architecture presents several challenges that organizations must navigate to ensure successful implementation. Framework for Modernizing Data Architecture To successfully modernize your data architecture, a well-defined framework is crucial. This structured approach minimizes disruption and ensures long-term value. Here’s a step-by-step guide: Best Practices for Data Architecture Modernization Implementing best practices during your data architecture modernization journey can help minimize risks and maximize the return on investment. Steps to Implement a Modern Data Architecture Implementing a modern data architecture requires careful planning and execution. By following a structured approach, organizations can ensure a smooth transition and successful implementation. 1. Define Business Goals and Data Strategy Before embarking on a modernization journey, it’s essential to define clear business goals and a data strategy. Understanding how your data architecture aligns with your broader business objectives will help guide the design and implementation of the new architecture. 2. Assess the Current Data Landscape A thorough assessment of your existing data systems and infrastructure is necessary to identify gaps, weaknesses, and opportunities for improvement. This assessment will inform the decisions you make regarding technology investments and integration efforts. 3. Plan for Integration and Interoperability Ensure that your new data architecture is designed for easy integration with existing systems. Focus on creating an interoperable environment that allows data to flow seamlessly across platforms. 4. Establish Security and Privacy Measures As data architectures evolve, it’s essential to implement robust security and privacy measures. This will ensure that sensitive data is protected and that your organization remains compliant with regulations. 5. Adopt a Scalable and Future-Proof Technologies Choose technologies that grow with your organization. Cloud-native solutions, containerization, and serverless architectures are great options for ensuring scalability and flexibility. These technologies allow you to respond to evolving demands without overhauling your infrastructure. 6. Implement Self-Serve Data Tools Provide teams with self-serve tools that enable them to access, analyze, and use data without heavy reliance on IT. Tools for data cataloging, visualization, and pipeline management can empower teams to derive insights independently, promoting a culture
Building a Data Mesh: Understanding and Choosing the Best One
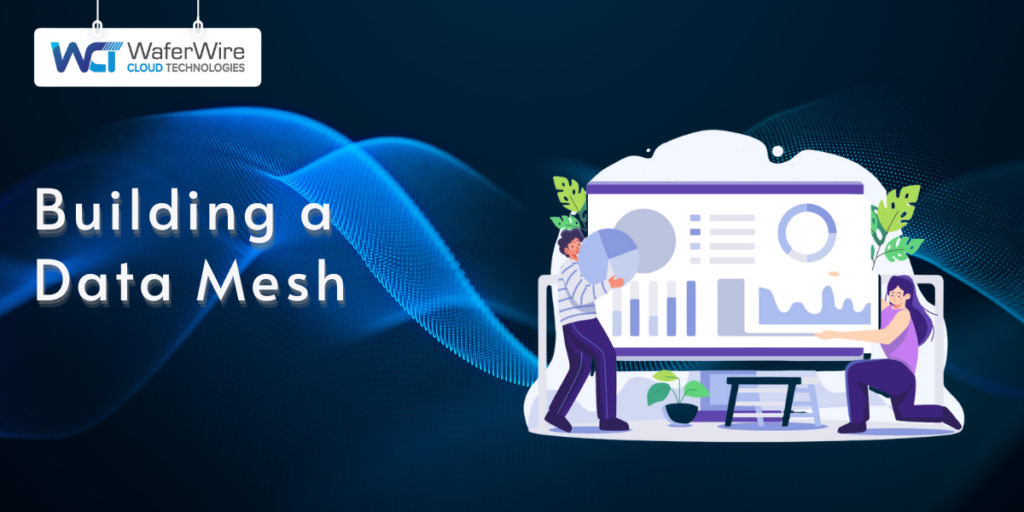
As businesses navigate an era of rapid technological advancement, the volume of data generated daily has reached unprecedented levels. Traditional centralized data management models, like data lakes, often fall short in meeting the growing need for agility and scalability. To address these limitations, organizations are adopting innovative approaches such as Data Mesh, enabling them to harness the full potential of their data assets. This blog will delve into the concept of Data Mesh, its guiding principles, the benefits it offers, and practical strategies for successful implementation. Understanding Data Mesh Before diving into the principles, let’s first understand what Data Mesh is and how it differs from traditional data architectures. Data Mesh is an architectural and organizational approach that shifts away from centralized data governance models, emphasizing decentralization and domain-oriented data ownership. Unlike traditional data architectures that rely heavily on centralized data teams and infrastructure, Data Mesh empowers individual domain teams to act as both data producers and consumers. By treating data as a product, organizations can foster a culture of ownership, accountability, and innovation, leading to more agile and scalable data solutions. This decentralized approach provides an alternative to the limitations of monolithic data lakes, offering greater flexibility and alignment with business objectives. A Decentralized Approach to Data Ownership and Management Traditional data systems often rely on centralized ownership, leading to inefficiencies and bottlenecks. Data Mesh addresses these challenges by decentralizing data ownership, empowering domain-specific teams to manage their data products. These teams are responsible for both producing and consuming data, ensuring it is relevant, accurate, and aligned with business objectives. A key feature of Data Mesh is its shift in perspective—treating data as a product rather than a byproduct. Unlike centralized data lakes, this approach emphasizes quality, usability, and lifecycle management. By placing accountability with the teams closest to the data, organizations can enhance accessibility, promote collaboration, and accelerate decision-making processes. Now that we’ve explored the fundamental aspects of Data Mesh, it’s important to understand the key principles that drive its success. Let’s take a closer look at these guiding principles. Key Principles of Data Mesh To ensure a successful implementation of Data Mesh, understanding the key principles behind it is crucial. These principles lay the foundation for the architecture and its successful integration within an organization. Decentralized Data Ownership Data Mesh places the responsibility of managing data in the hands of domain teams who have the most context about the data. This decentralized architecture reduces bottlenecks and ensures that data is managed effectively, efficiently, and in a way that directly supports business goals. Data as a Product Data Mesh emphasizes the need for teams to treat data as a product. This means focusing not just on the storage and access of data, but also on its quality, usability, and lifecycle management. This product mindset encourages teams to continuously improve their data products, ensuring they meet both current and future business needs. Self-Serve Data Infrastructure For Data Mesh to work effectively, domain teams need a standardized, self-serve data infrastructure. This means providing the right tools and platforms that make it easy for these teams to manage and analyze their data without requiring constant intervention from a central IT team. Self-service infrastructure improves efficiency and enables teams to be more agile in handling data needs. Federated Governance Data governance is an integral part of Data Mesh. Federated governance allows for centralized global standards while still maintaining local autonomy for domain teams. This approach ensures that data security, privacy, and compliance are upheld, but without compromising the flexibility of domain teams. With these key principles in mind, organizations can begin to realize the many benefits of implementing a Data Mesh architecture. Let’s explore how adopting Data Mesh can enhance your data management strategies. For expert guidance on building and optimizing your data strategies, explore our Data Strategy and Consulting services. Benefits of Implementing Data Mesh Adopting a Data Mesh approach offers numerous advantages that can significantly improve data management and drive organizational efficiency, especially for teams aiming to scale their data strategies effectively. While the benefits of Data Mesh are significant, successful implementation requires careful planning. In the next section, we will discuss the key considerations for organizations looking to implement Data Mesh. Implementing Data Mesh: Key Considerations Adopting a Data Mesh architecture can be transformative, but its success hinges on meticulous planning and execution. Here are some key considerations when moving forward with a Data Mesh architecture: Organizational Shifts To implement Data Mesh effectively, organizations must undergo structural changes. The first step is empowering domain teams with the autonomy to manage their own data. Additionally, roles like data product owners should be created to oversee the quality and management of data products within each domain. Tech Infrastructure Adopting Data Mesh requires the right technological infrastructure. This includes investing in automation tools and ensuring the use of interoperable standards to make data management across domains as seamless as possible. Technologies like cloud platforms, advanced analytics, and machine learning tools will be essential for managing data at scale. Cultural Change Data Mesh also requires a cultural shift within the organization. Teams must embrace a data-driven mindset and be willing to adopt new ways of working with data. Additionally, improving data literacy across the organization will be crucial for ensuring that teams can effectively manage their data and contribute to the overall success of the Data Mesh. Despite the many advantages of Data Mesh, the implementation process can come with its own set of challenges. Let’s look at some of the potential hurdles organizations may face when transitioning to this new model. Challenges and Considerations While the benefits of Data Mesh are clear, there are challenges to consider as well. Here are some of the main obstacles organizations may face during implementation: Once the foundational challenges are addressed, organizations must begin migrating their data to the new system. Let’s explore how this migration works and the best practices for successful conversion. Migrating to Data Products The migration to a Data Mesh architecture