In the age of big data, information has evolved from a mere resource to a powerful asset capable of transforming how businesses operate. Data plays a crucial role in customer insights, operational efficiency, and strategic decision-making.
However, the challenge organizations are facing today is not just how to collect data, but how to manage it effectively. When handled poorly, data can become chaotic and unreliable, making it more of a liability than an asset.
This is where effective data management comes in—ensuring data is accessible, accurate, secure, and compliant with evolving regulations. But the journey to effective management is not without obstacles.
The data management and analytics market is expected to expand at a Compound Annual Growth Rate (CAGR) of 16%, with a projected value of $513.3 billion by 2030. From overcoming data silos and inconsistencies to managing security risks, organizations face several hurdles.
Fortunately, proven strategies and best practices can help navigate these challenges and unlock the true value of data.
In this article, we’ll explore why effective data management is critical, the common challenges organizations face, and how adopting best practices can pave the path to data-driven success.
The Importance of Effective Data Management
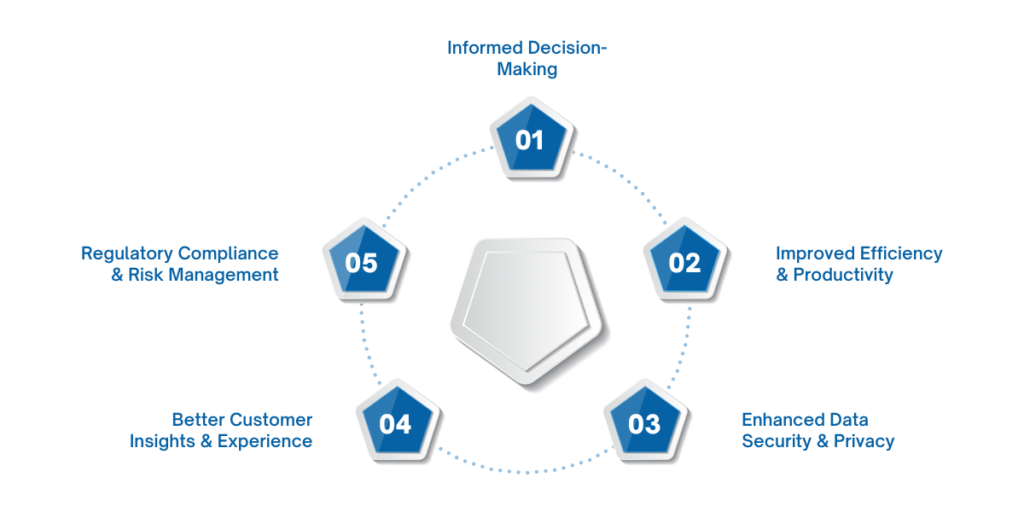
As data is generated at an unprecedented rate, managing it has become a necessity for organizations of all sizes. The value of data extends far beyond numbers or customer details; it powers innovation, decision-making, and business growth. Here’s why effective data management is essential:
Informed Decision-Making
Data-driven decisions provide a distinct advantage over assumptions or gut-feelings. With proper data management, organizations can ensure their data is accurate, timely, and relevant—leading to better strategic planning, improved forecasts, and quicker responses to market changes.
Improved Efficiency and Productivity
When data is organized and easily accessible, employees spend less time searching for information and more time using it effectively. Eliminating redundancies and minimizing data silos enhances collaboration across departments and increases overall productivity.
Enhanced Data Security and Privacy
With the rise of cyberattacks and stricter regulations (e.g., GDPR, CCPA), safeguarding sensitive data is more important than ever. Effective data management ensures proper protection through encryption, access controls, and secure storage, while helping organizations maintain compliance and avoid costly penalties.
Better Customer Insights and Experience
Effective data management enables businesses to analyze customer data, revealing behaviors, preferences, and pain points. This leads to personalized experiences, targeted marketing, and improved products/services, fostering stronger customer relationships and loyalty.
Regulatory Compliance and Risk Management
Navigating the complex landscape of data privacy and security regulations can be challenging. However, effective data management ensures that data is stored, accessed, and used in accordance with legal requirements, minimizing the risk of penalties and legal issues.
As organizations recognize the significance of these benefits, it becomes clear that effective data management is not just a technical necessity, but a strategic imperative. However, despite its importance, organizations face a range of challenges when it comes to managing their data effectively.
Challenges in Data Management
Despite its importance, effective data management comes with its share of challenges. As organizations handle increasing amounts of data from diverse sources, managing and leveraging that data becomes more complex. Here are some common obstacles:
- Data Overload: The volume of data being generated today can be overwhelming. Without proper systems, it becomes difficult to make sense of the data and extract useful insights. Effective data management involves organizing and structuring this vast amount of information to make it accessible and valuable.
- Data Silos: Data silos—where information is isolated in different departments or systems—can create inconsistencies and hinder collaboration. Without a unified view, it’s challenging to make comprehensive, informed decisions.
- Data Quality Issues: Poor data quality, including inaccuracies, missing values, and outdated information, can lead to misguided decisions and missed opportunities. Regular data cleansing and maintenance are crucial to ensure data remains reliable and useful.
- Data Security and Privacy Concerns: With the rise in cyber threats and stringent privacy regulations, data security and compliance are top priorities. Protecting sensitive data from unauthorized access while adhering to legal requirements is a growing challenge for organizations.
Addressing these challenges requires a systematic approach that encompasses key data management processes, ensuring that data is managed efficiently and securely. With these processes in place, organizations can overcome hurdles and realize the full potential of their data.
Also Read: 7 Essential Cost-Effective Data Management Techniques
Key Data Management Processes
Effective data management requires the implementation of various processes that ensure data is collected, organized, stored, and used in a manner that maximizes its value while minimizing risks. These processes help ensure data quality, security, and compliance, and enable organizations to leverage data for better decision-making and operational efficiency. Here are the key data management processes that are essential for any organization:
Data Collection and Acquisition
The first step in data management is the collection or acquisition of data from various sources. Whether it’s customer data, transactional data, or external data streams, gathering accurate and relevant information is critical.
Key Activities:
- Source Identification: Identifying where data will come from (e.g., sensors, databases, customer surveys, third-party vendors).
- Data Entry: Ensuring that data is entered correctly and consistently across systems.
- Data Integration: Integrating data from multiple sources into a unified system or database for analysis.
Challenges:
- Ensuring data is accurate and complete at the point of collection.
- Avoiding the collection of irrelevant or redundant data.
Data Storage and Organization
Once data is collected, it needs to be stored in a structured, organized manner to make it accessible and easy to manage. Effective data storage strategies allow organizations to quickly retrieve, update, and process data as needed.
Key Activities:
- Database Design: Structuring databases for easy storage, retrieval, and scalability (e.g., relational databases, data lakes, cloud storage).
- Data Categorization: Classifying data according to predefined categories, such as sensitive versus non-sensitive, structured versus unstructured.
- Data Archiving: Storing older or less frequently used data in an archive to maintain system performance.
Challenges:
- Deciding on the right storage solution (e.g., on-premise vs. cloud).
- Ensuring that data remains organized and retrievable as volumes grow.
Data Quality Management
Ensuring that data is accurate, complete, and reliable is crucial for making informed decisions. Data quality management is the process of improving, maintaining, and validating data to ensure it meets organizational standards and is fit for use.
Key Activities:
- Data Profiling: Assessing data to understand its structure, quality, and completeness.
- Data Cleansing: Correcting or removing inaccurate, incomplete, or outdated data.
- Data Standardization: Ensuring that data follows consistent formats across all sources (e.g., standardizing date formats, units of measurement).
- Data Validation: Implementing processes and rules to ensure data is accurate when entered or updated.
Challenges:
- Identifying and fixing data quality issues, especially when dealing with large volumes of data.
- Maintaining data quality over time as data evolves.
Data Integration
Data integration is the process of combining data from different sources and systems to create a unified, comprehensive dataset. It ensures that all data is aligned and accessible for analysis, reporting, and decision-making.
Key Activities:
- ETL (Extract, Transform, Load): Extracting data from multiple sources, transforming it into a consistent format, and loading it into a central database or data warehouse.
- Data Linking: Ensuring that data from different systems is connected, eliminating redundancies and ensuring a single version of the truth.
- Data Transformation: Converting data into a usable format for different systems or applications.
Challenges:
- Dealing with data from disparate systems, often with different formats, standards, and structures.
- Managing real-time data integration as businesses require immediate access to up-to-date data.
Data Security and Privacy Management
Data security and privacy management are critical to protecting sensitive data from unauthorized access, breaches, and ensuring compliance with regulations (such as GDPR or CCPA). This process ensures that data remains safe, while also ensuring compliance with data protection laws.
Key Activities:
- Data Encryption: Protecting sensitive data by converting it into an unreadable format for unauthorized users.
- Access Control: Implementing role-based access controls to restrict data access based on user roles.
- Backup and Recovery: Ensuring that data is regularly backed up and can be recovered in case of system failure or disaster.
- Compliance Management: Regularly reviewing data policies to ensure they comply with privacy laws and industry regulations.
Challenges:
- Keeping up with evolving data security threats and implementing the latest protective measures.
- Balancing the need for secure data with the need for easy access by authorized users.
By integrating these key processes into their operations, organizations can ensure their data is accurate, secure, and utilized effectively. But even with these systems in place, it is crucial to adopt best practices for maintaining and optimizing data management.
Best Practices for Data Management
To ensure data is accurate, secure, and accessible for decision-making, organizations should follow best practices in data management. These practices help maintain high-quality, secure, and reliable data:
- Establish Clear Data Governance: Data governance defines who owns, manages, and is responsible for data within an organization. Clear data governance ensures consistency, accountability, and compliance with regulations, helping organizations make the most of their data assets.
- Focus on Data Quality Management: High-quality data is the foundation of effective decision-making. Regularly clean, standardize, and validate data to maintain its accuracy and reliability. Use automated tools to streamline these processes and ensure data consistency.
- Implement Robust Data Security Measures: Data security is vital to protecting sensitive information from breaches and unauthorized access. Encrypt data, implement role-based access controls, and conduct regular security audits to minimize risks.
- Ensure Data Integration and Interoperability: Integrate data from various sources to create a unified, comprehensive dataset. Use ETL tools, standardized formats, and APIs to ensure smooth data integration and eliminate silos.
- Use Data Analytics for Decision-Making: Leverage data analytics to gain actionable insights and make data-driven decisions. Use descriptive, predictive, and real-time analytics to optimize business operations and improve customer experiences.
These best practices, when implemented effectively, provide a solid framework for managing data in a way that supports business goals and ensures ongoing value. Organizations should also adopt the right tools and techniques to further streamline their data management efforts.
Also Read: Top Data Integration Tools and Techniques
Data Management Tools and Techniques
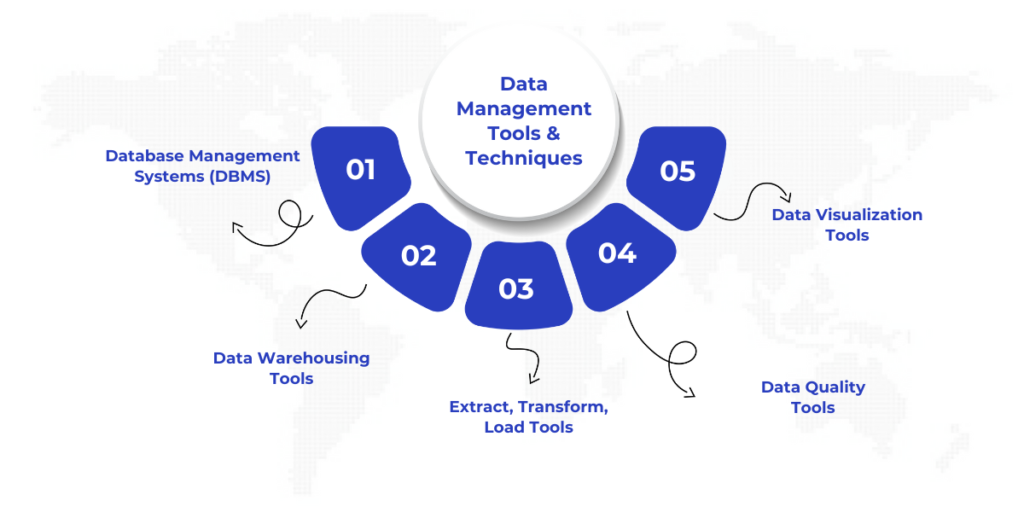
To streamline data management, organizations use various tools and techniques to ensure data remains accurate, secure, and easily accessible. Some widely used tools include:
- Database Management Systems (DBMS): DBMS tools such as MySQL, PostgreSQL, and Oracle provide structured data storage, quick retrieval, and support for complex queries, ensuring efficient data management.
- Data Warehousing Tools: Tools like Amazon Redshift and Google BigQuery centralize large datasets for easy access and advanced analytics, enabling organizations to generate insights from historical data.
- Extract, Transform, Load Tools: ETL tools like Apache Nifi and Talend automate data extraction, transformation, and loading, simplifying the integration and cleaning of data from multiple sources.
- Data Quality Tools: Data quality tools like Trifacta and Informatica ensure that data is clean, consistent, and accurate, enabling organizations to maintain high-quality datasets for analysis.
- Data Visualization Tools: Tools like Tableau and Power BI help organizations present data in an easily understandable format, making it easier for decision-makers to interpret insights and take action.
With the right tools and techniques in place, organizations can significantly enhance their data management practices, making it easier to make data-driven decisions.
Conclusion
Effective data management empowers organizations to make informed decisions, improve operational efficiency, and better serve their customers, all while mitigating risks and ensuring compliance. In a world where data is a valuable resource, mastering its management is the key to driving sustained growth and success.
Ready to take control of your data management and unlock its full potential? At WaferWire, we specialize in helping businesses streamline their data processes, ensure data security, and drive informed decision-making. Our expert solutions and tools are designed to optimize your data management strategy and support your business growth.
Contact us today to learn how we can help you transform your data into a valuable asset!