Data is the lifeblood of modern businesses, but managing it effectively can feel overwhelming. On one side, there’s the flexibility of data lakes—great for storing massive amounts of unstructured data. On the other, data warehouses excel in providing structured data for fast analytics. But what if you didn’t have to choose? Enter the data lakehouse, a powerful hybrid solution that combines the scalability of data lakes with the analytical performance of data warehouses.
In this article, we’ll explore how data lakehouses solve common pain points, such as siloed data, slow query speeds, and the high costs of maintaining separate systems.
What is a Data Lakehouse?
A data lakehouse combines the best of two worlds—data lakes and data warehouses—into one unified platform. It is designed to handle the flexibility and scale of unstructured data from data lakes while also delivering the performance and structure of data warehouses. This innovation eliminates the need to choose between the two, giving businesses a single solution to store, process, and analyze data.
Unlike traditional approaches, a data lakehouse supports both structured data (sales reports) and unstructured data (video files or social media feeds). This enables organizations to consolidate their analytics and big data processing into one platform. It’s a game-changer for industries that require high-speed analysis of diverse data types, such as retail, healthcare, and financial services.
Data Lake vs Data Warehouse
A data lake is a centralized repository that stores huge amounts of raw data in its original format, such as structured, semi-structured, and unstructured data. It enables businesses to store data as-is without first structuring it, giving them more freedom to use it later.
In contrast, a data warehouse is a system that aggregates structured data from multiple sources into a single central repository. This data is typically cleaned, transformed, and organized to support business intelligence activities, such as reporting and data analysis, facilitating informed decision-making.
With a clear understanding of data lakehouse, we can now delve into the essential benefits and features that position it as a game-changing solution for contemporary businesses.
Benefits of a Data Lakehouse
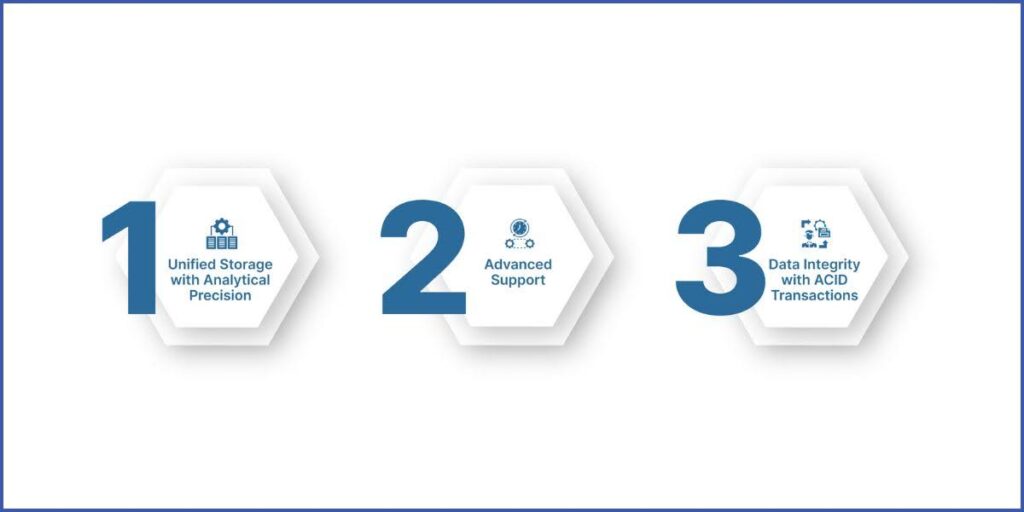
A data lakehouse revolutionizes data management by combining data lakes’ flexibility with data warehouses’ analytical power. Beyond simplifying workflows, it offers distinct benefits that drive business value.
- Unified Storage with Analytical Precision: A data lakehouse reduces expenses and streamlines management by combining organized and unstructured data into a single, accessible platform, eliminating the need for several systems. For example, a healthcare provider can store patient records alongside diagnostic images, enabling seamless analytics for improved outcomes.
- Advanced Support: Data lakehouses combine disparate datasets, providing a solid platform for machine learning algorithms. Analysts can train algorithms using clean, organized data, resulting in more accurate predictions. This might imply using consumer purchase histories and social media trends to improve recommendation engines in the retail industry.
- Data Integrity with ACID Transactions: ACID transactions ensure every data operation remains consistent and secure. This feature is especially valuable for industries like finance, where accurate and compliant record-keeping is critical. For instance, a bank can process millions of transactions while maintaining error-free reports for audits and regulations.
Having understood data lakes’ unique capabilities, it’s time to uncover the key technologies driving their evolution and transforming modern data management.
Key Technologies Enabling Data Lakehouses
The innovation of a data lakehouse relies on advanced technologies that bridge the gap between raw data storage and structured analytics.
1. Metadata Layers for Schema Enforcement
Metadata layers enforce schemas and validate data integrity, ensuring consistency and accuracy in data management. They also act as the backbone of governance, making it easier to track, catalog, and organize diverse datasets. For example, retail companies use metadata layers to unify product data and customer records, simplifying inventory analysis and personalized marketing.
2. High-Performance Query Engines
Modern query engines bring SQL capabilities to data lakes, enabling faster and more efficient queries. It converts raw data into useful insights without requiring complicated operations. Industries like financial services rely on these engines to process real-time analytics, such as fraud detection or risk management.
These foundational technologies set the stage for a deeper understanding of a data lakehouse’s operation. Let’s now examine the architecture that powers this transformative approach, from data ingestion to metadata management.
Data Lakehouse Architecture
A data lakehouse architecture forms the backbone of modern data management. Its structured layers seamlessly integrate to provide high performance, scalability, and robust governance, enabling businesses to unlock the full potential of their data.
- Ingestion Layer: The ingestion layer captures data from diverse structured, semi-structured, or unstructured sources. It processes raw information and converts it into formats ready for analysis. This capability supports real-time applications such as IoT device monitoring and customer behavior analysis, ensuring actionable insights are always within reach.
- Storage Layer: At the heart of a data lakehouse, the storage layer accommodates all data types using advanced formats like Parquet and ORC. It ensures durability, reliability, and easy access to stored data. Additionally, its scalability reduces costs while meeting the demands of industries with massive datasets, such as healthcare and manufacturing.
- Metadata Layer: The metadata layer organizes and catalogs data, enabling seamless search, governance, and lineage tracking. It ensures compliance with regulations like GDPR and helps businesses manage their data assets more effectively. For instance, utility companies can use this layer to monitor operational data across multiple sites.
With a clear understanding of the data architecture, let’s explore its real-world applications and how it drives value across industries.
Use Cases and Applications
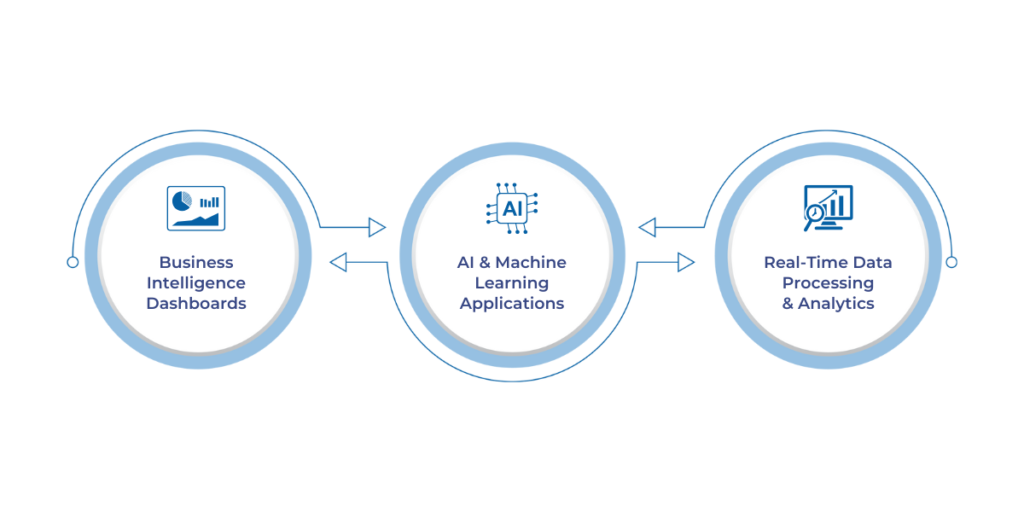
The versatility of a data lakehouse makes it indispensable for modern businesses, supporting everything from operational analytics to advanced AI solutions.
1. Business Intelligence Dashboards
Data lakehouses provide a strong foundation for BI dashboards, enabling businesses to analyze vast amounts of structured and unstructured data in real-time. As a result, it allows organizations to generate meaningful insights, optimize decision-making, and enhance reporting capabilities.
2. AI and Machine Learning Applications
AI-driven solutions, such as bots and virtual assistants, utilize the scalable storage and processing capabilities of data lakehouses. Businesses can build intelligent chatbots, automate customer service, and enhance predictive analytics, improving operational efficiency and user engagement.
3. Real-Time Data Processing and Analytics
Industries like healthcare and manufacturing rely on real-time analytics for critical operations. A data lakehouse supports immediate data processing, such as monitoring patient vitals or analyzing production line efficiency.
Beyond supporting operations, a data lakehouse excels in managing data integrity and governance. Here’s how it ensures trustworthiness and compliance.
Data Management and Governance
Strong data management and governance lie at the heart of every data lakehouse, building trust and enabling smarter decisions across industries.
- Guarantees High-Quality Data: Standardized governance protocols, such as automated data validation and consistency checks, ensure reliable and complete information. For instance, a retailer can avoid inventory mismatches by maintaining consistent product data and improving both analytics and operations.
- Enhances Transparency with Data Lineage: A robust metadata layer maps the data flow across systems, providing clarity and ensuring compliance with regulations like GDPR. In retail, tracking the lineage of customer purchase data enables businesses to create targeted campaigns while adhering to privacy laws.
Conclusion
The rapid growth of data demands solutions that are as flexible as they are powerful. A data lakehouse simplifies data management and empowers businesses to unlock insights with unparalleled speed and accuracy. Combining the scalability of data lakes with the analytical precision of data warehouses creates a single, unified platform for all your data needs.
However, technology is only as effective as its implementation. That’s where WaferWire steps in. As a trusted partner in digital transformation, our team goes beyond providing tools; it delivers tailored solutions that align with your unique business goals.
Don’t let outdated systems hold you back. Take the first step toward a smarter, more agile data strategy. Contact us today to explore how a data lakehouse can revolutionize your operations, enhance decision-making, and position your business for long-term success.