According to 2025 Gartner CIO and Technology Executive Survey, only 48% of digital initiatives meet or exceed their business outcome targets. This highlights the challenges organizations face in achieving successful digital transformations.
Microsoft Fabric Notebooks provide a powerful solution for overcoming these challenges. By offering a flexible platform for data ingestion, they enable data engineers and analysts to easily import and process data. These notebooks bridge the gap between raw information and actionable insights, enabling organizations to transform their data into a valuable asset that drives smarter business decisions.
With Fabric Notebooks, users can:
- Manage both batch and real-time data pipelines.
- Seamlessly integrate with Fabric’s Lakehouse architecture for optimized data storage and processing.
- Leverage the power of Python and Scala within a secure Microsoft ecosystem, offering flexibility in coding.
From retailers processing millions of daily transactions to healthcare organizations unifying patient records, Fabric Notebooks help transform data chaos into structured value.
This guide will walk you through how Fabric Notebooks simplify data ingestion, focusing on core features, real-world use cases, and best practices for efficient data management.
What is Data Ingestion in Fabric
Data ingestion is the process of importing data from various sources into a system, such as Microsoft Fabric, to be analyzed and processed. This process is crucial for transforming raw data into actionable insights that businesses can utilize to make informed decisions.
Why use Notebooks for ingestion?
Fabric Notebooks provide a flexible, code-first approach to ingesting data, enabling users to work directly with data using programming languages such as Python and Scala. This flexibility enables easy customization of ingestion workflows and optimization for specific business needs. Fabric Notebooks allow engineers and analysts to take complete control over the ingestion process, ensuring data is prepared and processed efficiently.
Key Scenarios: Batch vs. Streaming, Structured vs. Unstructured Data
Batch data ingestion involves processing large volumes of data at once. It is ideal for scenarios where data does not need to be real-time, such as historical data analysis.
Streaming data ingestion, on the other hand, processes data in real time, making it ideal for scenarios that require immediate analysis, such as fraud detection in finance or monitoring customer activity on e-commerce sites.
With an understanding of data ingestion, the next step is setting up the Fabric Portal environment. Let’s walk through how you can prepare your Microsoft Fabric workspace for seamless data ingestion.
Setting Up Your Fabric Environment
Getting started with Fabric Notebooks begins with setting up your Microsoft Fabric environment. This is a critical first step in ensuring smooth data ingestion and processing. The right setup ensures that all the tools you need are ready and integrated to your workflows.
Prerequisites:
- Access to Microsoft Fabric: You will need either a trial or licensed version of Microsoft Fabric to get started.
- Basic familiarity with Python/Scala: Since Fabric Notebooks allow you to write custom code, a basic understanding of Python or Scala will help you get the most out of the platform. These languages provide the flexibility you need for complex data manipulation and processing.
Navigate the Fabric Portal: Locate Data Engineering Workspace
Once you have access, the next step is to log into the Fabric Portal. Here’s where you will find all your data engineering tools:
- Go to the Data Engineering workspace in the Fabric Portal. This is where you can manage your data pipelines and notebooks.
- You’ll be able to set up new Fabric Notebooks and begin creating your data ingestion workflows, whether you are dealing with batch or streaming data.
With your environment ready, it’s time to create and configure your first Fabric Notebook. Let’s explore the steps for building a notebook that fits your data ingestion needs.
Creating and Configuring a Notebook
Getting started with Fabric Notebooks involves setting up a notebook tailored to your data ingestion needs. Whether you’re processing batch data or dealing with real-time streams, it offers the flexibility and control to build robust data workflows.
Step-by-Step Guide for Setting Up Fabric Notebook
1. Launch a New Notebook from the Fabric Portal
Start by opening the Fabric Portal. Navigate to the Data Engineering workspace and create a new notebook. This will serve as the foundation for your data ingestion tasks.
2. Choose Your Kernel (Python/Scala)
Fabric Notebooks allow you to choose between Python and Scala as your programming language. Both offer flexibility, with Python being widely used for data analysis and Scala for high-performance data processing. Choose the one that best suits your needs or the expertise of your team.
3. Attach a Lakehouse as a Storage Target
The next step is to attach a Lakehouse to your notebook as the storage target. This is essential for ensuring that the data you ingest gets stored and processed efficiently within the Fabric environment. The Lakehouse architecture integrates data lakes and data warehouses for improved accessibility and scalability.
Now that your notebook is set up, let’s dive into the different data ingestion methods available in Fabric Notebooks. We will examine how you can efficiently integrate data into the system using various approaches tailored to your specific needs.
Data Ingestion Methods
Ingesting data into Microsoft Fabric is essential for transforming raw information into actionable insights. Fabric Notebooks offer a flexible, code-first approach to data ingestion, making it easier for data engineers and analysts to bring in data from various sources. Depending on the type of data you are working with, Fabric Notebooks offer various methods for efficient ingestion. Let’s take a closer look at the primary data ingestion methods:
A. Import from Files (CSV, JSON, Parquet)
One of the simplest and most common ways to ingest data is by importing files such as CSV, JSON, or Parquet. These formats are widely used for storing structured data and are often used in batch processing.
- Code Snippets:
- To load CSV data:
pandas.read_csv() - For JSON files:
spark.read.json() - To read Parquet:
spark.read.parquet()
- To load CSV data:
- Real-World Example:
Imagine a retail business needs to process sales data stored in a CSV file on blob storage. Using the pandas.The read_csv() function in a Fabric Notebook allows for the quick ingestion and analysis of this data to track sales performance and adjust strategies in real time.
B. Connect to Databases (SQL, Cosmos DB)
In addition to file imports, Fabric Notebooks allow users to connect to databases for more structured data. Whether you are using traditional SQL databases or modern databases like Cosmos DB, Fabric Notebooks makes connecting and pulling data a straightforward process.
- Using JDBC/ODBC Drivers:
To connect to a database, you can use JDBC or ODBC drivers within your notebook to query databases and pull records. - Example:
A financial institution can use this method to connect to an Azure SQL Database, pull real-time customer data, and use it to monitor for unusual activity or potential fraud.
C. Stream Real-Time Data (Kafka, Event Hubs)
For businesses that need real-time data insights, Fabric Notebooks support streaming data ingestion using technologies such as Kafka or Event Hubs. Structured Streaming in PySpark enables you to process data as it arrives, allowing for immediate analysis and informed decision-making.
- Mini-lab Example:
Suppose you have a fleet of IoT devices sending real-time telemetry data. By connecting Fabric Notebooks to a Kafka stream, you can ingest the data and monitor device performance instantly, providing immediate feedback to improve operational efficiency.
Transform ingested data into actionable insights using Fabric Notebooks.
Transforming Data During Ingestion
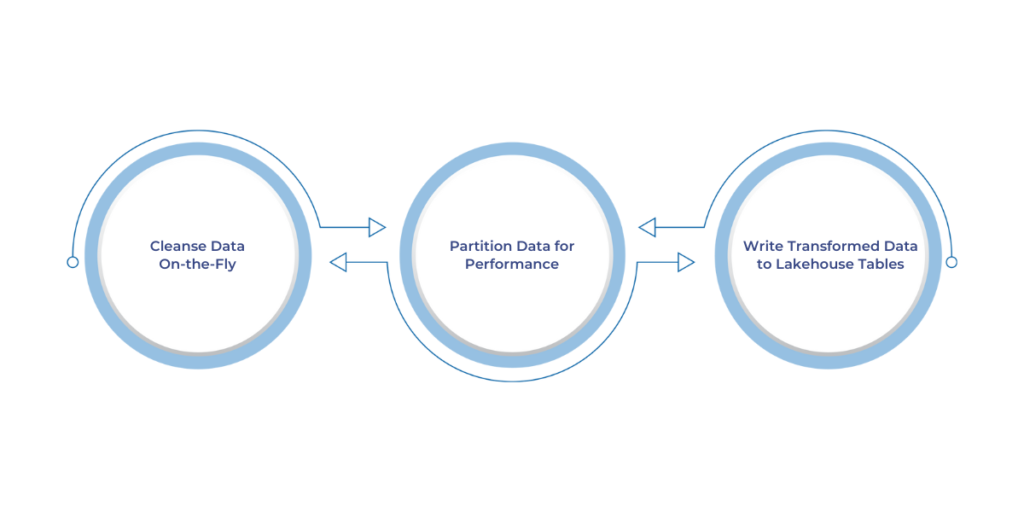
Fabric Notebooks offer a streamlined approach to handling and preparing data for further analysis, ensuring it is clean, optimized, and ready for use. With the right transformation steps, you can provide your data that is both accurate and efficient for downstream processes.
Cleanse Data On-the-Fly
During data ingestion, it’s essential to cleanse the data, handling issues such as null values, duplicates, or inconsistent formats. This process helps maintain the integrity of your datasets, ensuring that what enters your Lakehouse is clean and reliable. By using simple code in Fabric Notebooks, you can eliminate problematic data points before they affect your analysis.
Partition Data for Performance
Partitioning data based on relevant attributes, like date, can significantly improve performance when querying and analyzing large datasets. By segmenting your data, you can ensure that queries are more efficient and that the system only processes the necessary portions of the data. This is crucial for working with large, time-sensitive datasets where speed is important.
Write Transformed Data to Lakehouse Tables
Once the data is cleansed and partitioned, it’s time to store it in your Lakehouse tables. This ensures that the data is accessible for future use and analysis. The Fabric Notebooks environment allows you to save the processed data directly to Lakehouse tables, making it easy to link the ingested data with the rest of your organization’s data infrastructure.
With the data properly cleansed, partitioned, and stored, the next step is to monitor and troubleshoot. Let’s explore how you can ensure your data pipelines are running smoothly, identify potential issues, and get the support you need.
Monitoring and Troubleshooting
As with any data processing system, monitoring and troubleshooting are essential to ensure smooth operations in Fabric Notebooks. Keeping an eye on your workflows and promptly resolving issues ensures that data pipelines run efficiently and effectively.
Track Job History in Fabric Portal
One of the key features in Fabric Notebooks is the ability to track job history directly in the Fabric Portal. This allows you to monitor the status of each job, check if it ran successfully, and review any errors or issues that might have occurred. By analyzing job history, you can pinpoint bottlenecks or failures in your data workflows and take proactive steps to address them.
Debug Common Errors (Timeouts, Permission Issues)
Common issues like timeouts or permission errors can often disrupt data ingestion and processing. With Fabric Notebooks, you can quickly debug these problems by reviewing detailed error logs. For example:
- Timeouts: If a data pipeline takes too long to process, check for performance issues or resource allocation problems.
- Permission Issues: Ensure that the correct roles and permissions are assigned in the Fabric Portal to allow necessary access to the data.
With these tools, troubleshooting becomes more straightforward, minimizing downtime and ensuring smooth data operations.
With monitoring and troubleshooting in place, it’s time to explore how you can further optimize your data workflows. Let’s dive into transforming data during ingestion to ensure that your data is clean and accurate.
Best Practices
When working with Fabric Notebooks, it’s essential to follow best practices that ensure your data workflows are secure, efficient, and well-documented. By applying these practices, you can streamline your operations, reduce costs, and improve collaboration across teams.
Security: Use Short-Lived Credentials
Security is a top priority when handling sensitive data. One of the best practices is to use short-lived credentials, which expire after a set period. This reduces the risk of unauthorized access to your data. By limiting the time a user or application has access, you increase security without sacrificing workflow efficiency.
Cost Control: Auto-Pause Notebooks After Idle Time
To control costs, especially in cloud-based environments, enabling auto-pause for Fabric Notebooks is a simple yet effective solution. When notebooks are not in use, they automatically pause, preventing unnecessary resource consumption. This feature helps businesses manage their cloud costs by ensuring resources are only being used when necessary.
Pro Tip: Set auto-pause for non-critical data processes, especially for large enterprises that use Fabric Notebooks for batch processing. This ensures your cloud budget is used effectively without over-provisioning.
Documentation: Add Markdown Cells to Explain Steps
Clear documentation is crucial for maintaining a smooth workflow, particularly in collaborative environments. Using Markdown cells within your Fabric Notebooks allows you to document each step, ensuring that your notebooks are easy to understand for other team members. This practice reduces errors and accelerates onboarding for new team members.
Conclusion
Microsoft Fabric Notebooks are more than just a tool for data ingestion; they are a bridge to turning raw data into meaningful insights that directly impact your bottom line. Whether you’re streamlining inventory processes, speeding up financial reporting, or unifying healthcare records, these notebooks put you in control of your data, no matter how complex.
But here’s the thing: you can’t just use any solution. You need one that adapts to your needs, one that grows with you as your business scales and changes. With Fabric Notebooks, you get a flexible, code-first environment that does more than just ingest data. It’s designed to streamline workflows and enable you to act on your data faster and more effectively.
At WaferWire, we don’t just help you set up Fabric Notebooks; we make sure they’re tailored to your business needs. We help you:
- Build data pipelines that fit your specific industry needs
- Implement secure, scalable workflows that handle the data of tomorrow
- Optimize performance from day one so you can focus on the big picture
Are you prepared to revolutionize your business’s data management? Let’s discuss creating a data strategy that goes beyond the present and positions you for the future.